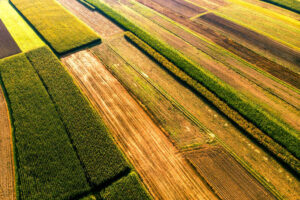

(A) Right mediolateral oblique unadulterated mammogram shows an 8-mm ill-defined mass (arrowhead), which, after biopsy, was determined to be a histologic grade 2 ductal carcinoma of no special type. (B) Mammogram shows findings by human readers (blue areas) and the Lunit INSIGHT MMG artificial intelligence (AI) algorithm (red cross). Each blue dot is a mark placed by an individual human reader on a perceived abnormality when the Personal Performance in Mammographic Screening (PERFORMS) case was read. A region of interest (pentagon) has been annotated by the PERFORMS scheme organizers and their expert radiology panel. AI has correctly marked the region of interest in the right breast for recall. Source: PERFORMS via Yan Chen.
Credit: Radiological Society of North America
Using a standardized assessment, researchers in the UK compared the performance of a commercially available artificial intelligence (AI) algorithm with human readers of screening mammograms. Results of their findings were published in Radiology, a journal of the Radiological Society of North America (RSNA).
Mammographic screening does not detect every breast cancer. False-positive interpretations can result in women without cancer undergoing unnecessary imaging and biopsy. To improve the sensitivity and specificity of screening mammography, one solution is to have two readers interpret every mammogram.
According to the researchers, double reading increases cancer detection rates by 6 to 15% and keeps recall rates low. However, this strategy is labor-intensive and difficult to achieve during reader shortages.
“There is a lot of pressure to deploy AI quickly to solve these problems, but we need to get it right to protect women’s health,” said Yan Chen, Ph.D., professor of digital screening at the University of Nottingham, United Kingdom.
Left mediolateral oblique mammogram. Unadulterated mammogram shows an asymmetric density (arrowhead) which, after biopsy, was determined to be a histologic grade 2 ductal carcinoma. (B) Artificial intelligence (AI) has correctly marked the region of interest in the left breast for recall (red cross) when set at a recall threshold of 2.91 or higher to match average human specificity, demonstrating a true-positive case. (C) AI has not marked the region of interest in the same breast when set at a recall threshold of 3.06 or higher, indicating a false-negative case. Blue dots indicate findings identified by the human readers. This shows how modifying the threshold for recall can impact the sensitivity of the AI model. Source: Personal Performance in Mammographic Screening via Yan Chen. Credit: Radiological Society of North America
Prof. Chen and her research team used test sets from the Personal Performance in Mammographic Screening, or PERFORMS, quality assurance assessment utilized by the UK’s National Health Service Breast Screening Program (NHSBSP), to compare the performance of human readers with AI. A single PERFORMS test consists of 60 challenging exams from the NHSBSP with abnormal, benign and normal findings. For each test mammogram, the reader’s score is compared to the ground truth of the AI results.
“It’s really important that human readers working in breast cancer screening demonstrate satisfactory performance,” she said. “The same will be true for AI once it enters clinical practice.”
The research team used data from two consecutive PERFORMS test sets, or 120 screening mammograms, and the same two sets to evaluate the performance of the AI algorithm. The researchers compared the AI test scores with the scores of the 552 human readers, including 315 (57%) board-certified radiologists and 237 non-radiologist readers consisting of 206 radiographers and 31 breast clinicians.
“The 552 readers in our study represent 68% of readers in the NHSBSP, so this provides a robust performance comparison between human readers and AI,” Prof. Chen said.
Treating each breast separately, there were 161/240 (67%) normal breasts, 70/240 (29%) breasts with malignancies, and 9/240 (4%) benign breasts. Masses were the most common malignant mammographic feature (45/70 or 64.3%), followed by calcifications (9/70 or 12.9%), asymmetries (8/70 or 11.4%), and architectural distortions (8/70 or 11.4%). The mean size of malignant lesions was 15.5 mm.
No difference in performance was observed between AI and human readers in the detection of breast cancer in 120 exams. Human reader performance demonstrated mean 90% sensitivity and 76% specificity. AI was comparable in sensitivity (91%) and specificity (77%) compared to human readers.
“The results of this study provide strong supporting evidence that AI for breast cancer screening can perform as well as human readers,” Prof. Chen said.
Prof. Chen said more research is needed before AI can be used as a second reader in clinical practice.
“I think it is too early to say precisely how we will ultimately use AI in breast screening,” she said. “The large prospective clinical trials that are ongoing will tell us more. But no matter how we use AI, the ability to provide ongoing performance monitoring will be crucial to its success.”
Prof. Chen said it’s important to recognize that AI performance can drift over time, and algorithms can be affected by changes in the operating environment.
“It’s vital that imaging centers have a process in place to provide ongoing monitoring of AI once it becomes part of clinical practice,” she said. “There are no other studies to date that have compared such a large number of human reader performance in routine quality assurance test sets to AI, so this study may provide a model for assessing AI performance in a real-world setting.”
“Performance of a Breast Cancer Detection AI Algorithm Using the Personal Performance in Mammographic Screening Scheme.” Collaborating with Dr. Chen were Adnan G. Taib, B.M.B.S., Iain T. Darker, Ph.D., and Jonathan J. James, FRCR.
In 2023, Radiology is celebrating its 100th anniversary with 12 centennial issues, highlighting Radiology’s legacy of publishing exceptional and practical science to improve patient care.
Radiology is edited by Linda Moy, M.D., New York University, New York, N.Y., and owned and published by the Radiological Society of North America, Inc. (https://pubs.rsna.org/journal/radiology)
RSNA is an association of radiologists, radiation oncologists, medical physicists and related scientists promoting excellence in patient care and health care delivery through education, research and technologic innovation. The Society is based in Oak Brook, Illinois. (RSNA.org)
For patient-friendly information on breast cancer screening, visit RadiologyInfo.org.
Journal: Radiology
Subject of Research: People
Article Title: Performance of a Breast Cancer Detection AI Algorithm Using the Personal Performance in Mammographic Screening Scheme
Article Publication Date: 5-Sep-2023
Media Contact
Linda Brooks
Radiological Society of North America
lbrooks@rsna.org
Office: 630-590-7738