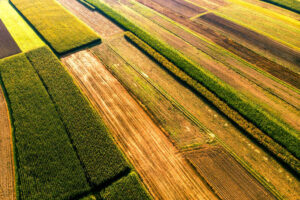
Machine Learning Models for Efficient Quantum Device Verification
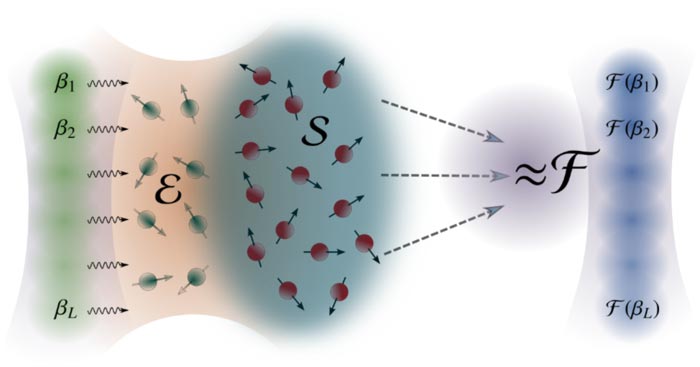
B and F represent the input and output states, respectively, of a quantum system. E is an auxiliary system necessary to pass the sequence of input states B to the quantum reservoir S. S can then be read to emulate F without disrupting the system.
Credit: ©2021 Tran et al.
A novel algorithm allows for efficient and accurate verification of quantum devices.
Technologies that take advantage of novel quantum mechanical behaviors are likely to become commonplace in the near future. These may include devices that use quantum information as input and output data, which require careful verification due to inherent uncertainties. The verification is more challenging if the device is time dependent when the output depends on past inputs. For the first time, researchers using machine learning dramatically improved the efficiency of verification for time-dependent quantum devices by incorporating a certain memory effect present in these systems.
Quantum computers make headlines in the scientific press, but these machines are considered by most experts to still be in their infancy. A quantum internet, however, may be a little closer to the present. This would offer significant security advantages over our current internet, amongst other things. But even this will rely on technologies that have yet to see the light of day outside the lab. While many fundamentals of the devices that can create our quantum internet may have been worked out, there are many engineering challenges in order to realize these as products. But much research is underway to create tools for the design of quantum devices.
Postdoctoral researcher Quoc Hoan Tran and Associate Professor Kohei Nakajima from the Graduate School of Information Science and Technology at the University of Tokyo have pioneered just such a tool, which they think could make verifying the behavior of quantum devices a more efficient and precise undertaking than it is at present. Their contribution is an algorithm that can reconstruct the workings of a time-dependent quantum device by simply learning the relationship between the quantum inputs and outputs. This approach is actually commonplace when exploring a classical physical system, but quantum information is generally tricky to store, which usually makes it impossible.
“The technique to describe a quantum system based on its inputs and outputs is called quantum process tomography,” said Tran. “However, many researchers now report that their quantum systems exhibit some kind of memory effect where present states are affected by previous ones. This means that a simple inspection of input and output states cannot describe the time-dependent nature of the system. You could model the system repeatedly after every change in time, but this would be extremely computationally inefficient. Our aim was to embrace this memory effect and use it to our advantage rather than use brute force to overcome it.”
Tran and Nakajima turned to machine learning and a technique called quantum reservoir computing to build their novel algorithm. This learns patterns of inputs and outputs that change over time in a quantum system and effectively guesses how these patterns will change, even in situations the algorithm has not yet witnessed. As it does not need to know the inner workings of a quantum system as a more empirical method might, but only the inputs and outputs, the team’s algorithm can be simpler and produce results faster as well.
“At present, our algorithm can emulate a certain kind of quantum system, but hypothetical devices may vary widely in their processing ability and have different memory effects. So the next stage of research will be to broaden the capabilities of our algorithms, essentially making something more general purpose and thus more useful,” said Tran. “I am excited by what quantum machine learning methods could do, by the hypothetical devices they might lead to.”
Journal article
Quoc Hoan Tran and Kohei Nakajima, “Learning Temporal Quantum Tomography”, Physical Review Letters, DOI: 10.1103/PhysRevLett.127.260401
Link: https://journals.aps.org/prl/abstract/10.1103/PhysRevLett.127.260401
Funding
This work is supported by MEXT Quantum Leap Flagship Program (MEXT Q-LEAP) Grant
Nos. JPMXS0118067394 and JPMXS0120319794.
Useful links
Graduate School of Information Science and Technology – https://www.i.u-tokyo.ac.jp/index_e.shtml
Physical Intelligence Lab – https://www.kohei-nakajima.com/
Next Generation Artificial Intelligence Research Center – https://www.ai.u-tokyo.ac.jp/en/
Research contact
Associate Professor Kohei Nakajima
Graduate School of Information Science and Technology, The University of Tokyo,
7-3-1 Hongo, Bunkyo-ku, Tokyo 113-8656, JAPAN
Email: k_nakajima@mech.t.u-tokyo.ac.jp
Press Contact
Mr. Rohan Mehra
Division for Strategic Public Relations, The University of Tokyo
7-3-1 Hongo, Bunkyo-ku, Tokyo 113-8654, JAPAN
Email: press-releases.adm@gs.mail.u-tokyo.ac.jp
About the University of Tokyo
The University of Tokyo is Japan’s leading university and one of the world’s top research universities. The vast research output of some 6,000 researchers is published in the world’s top journals across the arts and sciences. Our vibrant student body of around 15,000 undergraduate and 15,000 graduate students includes over 4,000 international students. Find out more at www.u-tokyo.ac.jp/en/ or follow us on Twitter at @UTokyo_News_en.
Journal: Physical Review Letters
DOI: 10.1103/PhysRevLett.127.260401
Method of Research: Computational simulation/modeling
Article Title: Learning Temporal Quantum Tomography
Article Publication Date: 22-Dec-2021