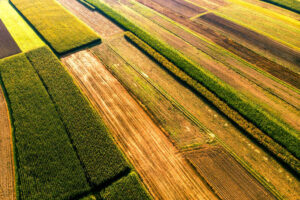
Uncovering Unique Structures with Big Data and Machine Learning
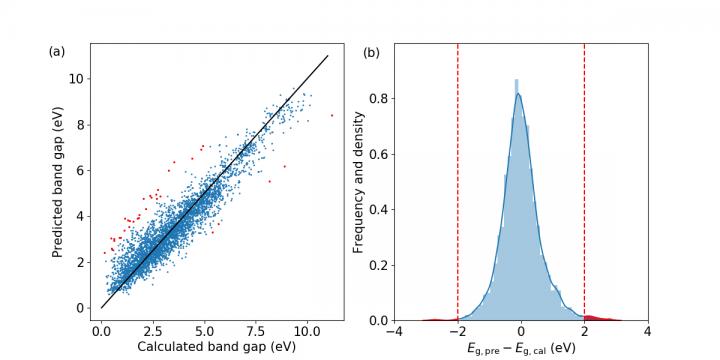
The machine learning results. (a) The scatter plot, and (b) the histogram of errors and the kernel density estimation of the probability density function. Red points and regions correspond to structures with prediction error larger than 2 eV.
Credit: ©Science China Press
While tremendously useful, these models do not directly show the rules and physics underlying the relationship between structure and property. And despite of their decent overall performance, there will always be some exceptions where ML models fail to give accurate predictions. Very often, it is these exceptions that shed some new insights about the underlying physics, and open up new frontiers in science.
A research group led by Prof. Feng Pan, the founding dean of the School of Advanced Materials, Peking University Shenzhen Graduate School, has recently shown that these models are valuable not only when they succeed in predicting properties accurately, but also when they fail.
In their work, a model is built to predict the HSE band gaps of compounds according to their atomic structures only, based on a high-throughput calculation database constructed by the school themselves.
The R2 of the model is 0.89, comparable with similar works. They then filtered out those structures with prediction error larger than 2 eV and examined them carefully.
Many structures with unusual structure units, or showing other abnormities with similar compounds, like relatively large band gaps or being in different phases. Among these unusual structures, AgO2F raises great interest and a detailed analysis is given.
It is found that Ag3+ and O22- coexist in this compound, and while Ag ions are in square planar coordination, there is little hybridization between orbitals of Ag and O. States near the band edges are mainly contributed by O-2p orbitals and the band gap is much smaller than other compounds with Ag3+ ions.
This offers a new example for anionic redox property, a hot topic in the investigation of Li-excess electrode materials. These results demonstrate how unusual structures can be discovered from exceptions in machine learning, which can help us to investigate new physics and novel structural units from existing databases.
###
Wang is supported by the Director, Office of Science (SC), Basic Energy Science (BES), Materials Science and Engineering Division (MSED), of the US Department of Energy (DOE) under Contract No.DE-AC02-05CH11231 through the Materials Theory program (KC2301). This work is also financially supported by National Key R&D Program of China (2016YFB0700600), Shenzhen Science and Technology Research Grant (ZDSYS201707281026184), and Guangdong Key-lab Project (2017B0303010130).
See the article:
Jianshu Jie, Zongxiang Hu, Guoyu Qian, Mouyi Weng, Shunning Li, Shucheng Li, Mingyu Hu, Dong Chen, Weiji Xiao, Jiaxin Zheng, Lin-Wang Wang and Feng Pan. Discovering unusual structures from exception using big data and machine learning techniques. Science Bulletin, 2019, 64(9)612-616; doi: 10.1016/j.scib.2019.04.015
https:/